
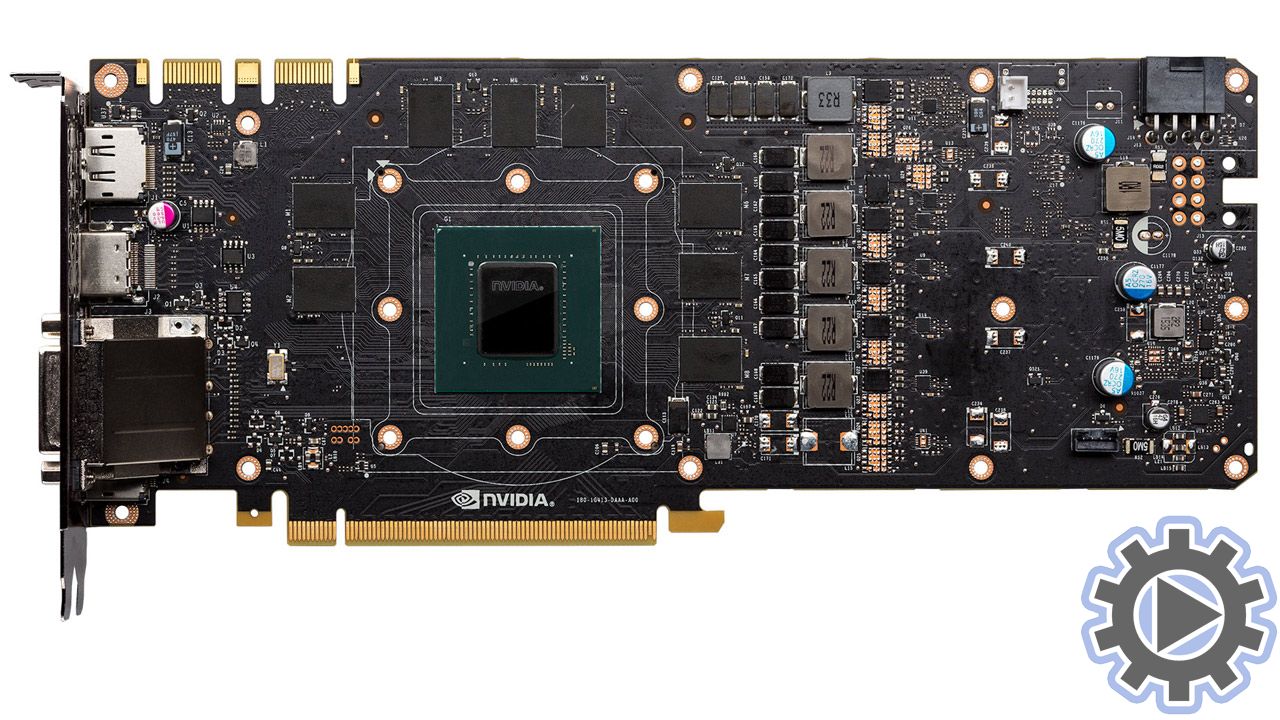
So we fine-tuned this model to adapt for our dataset and named it as 'Pondception'. However, this network performs very well on the ImageNet challenge, but not on the classes of interest here. The Inception v4 model is the best choice when selecting a model which has 95.2% Top-5 accuracy. Data is collected via aerial platforms, but at a view angle such that it resembles satellite imagery. The remaining datasets are 3-band RGB images. The Columbus and Vaihingen datasets are in grayscale. The dataset is described in detail by Mundhenk et al, 2016. The data consists of ~33,000 unique cars from six different image locales: Toronto Canada, Selwyn New Zealand, Potsdam and Vaihingen Germany, Columbus and Utah United States. The Cars Overhead with Context (COWC) dataset is a large, high quality set of annotated cars from overhead imagery. We have used the following components for the software: However, the cost of P100 is almost 15X more than the 1080 Ti. The direct CPU-to-GPU NVLink connectivity on P100 enables 5X faster transfers than standard PCI-E. Also, high-bandwidth HBM2 memory is significantly faster than GDDR5X.
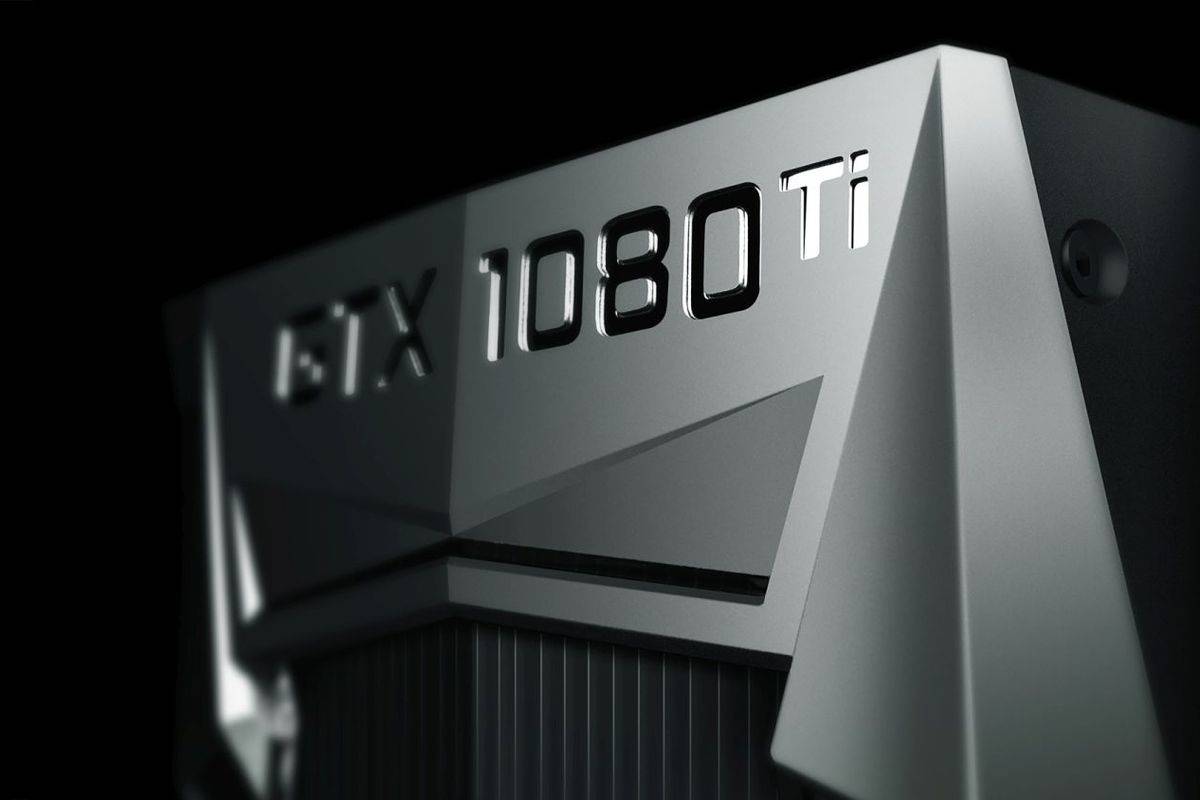
Tesla P100 has more memory than GTX 1080 Ti. The hardware specifications of both the devices are: The second is a Tesla P100 GPU, a high-end device devised for data centers which provides high-performance computing for Deep Learning. The first is a GTX 1080 Ti GPU, a gaming device. This whitepaper aims at comparing two different pieces of hardware that are often used for Deep Learning tasks. Performance Comparison between NVIDIA’s GeForce GTX 1080 and Tesla P100 for Deep Learning Introduction
